Сочетание подходов дистанционного зондирования и моделирования для оценки засоления почв орошаемых территорий бассейна Аральского моря
М. Ибрахимова*, У. Х. Аванб, М. Султанова, А. Акрамхановб, К. Джумабоевв, К. Конрадг,д, Д. Ламерсе
а Хорезмская cельская консультационная служба (KRASS), Ургенч, Хорезм, Узбекистан.
бМеждународный центр сельскохозяйственных исследований в засушливых регионах (ИКАРДА),Ташкент, Узбекистан.
вМеждународный институт управления водными ресурсами (IWMI), Региональный офис в Центральной Азии, Ташкент, Узбекистан.
гИнститут географии и геологии Вюрцбургского университета, Департамент дистанционного зондирования, Вюрцбург, Германия.
дУниверситет Галле, Институт геонаук и географии, Галле, Германия.
еЦентр исследований в области развития (ZEF), Департамент экологии и управления ресурсами,
Боннский университет, Бонн, Германия.
* для корреспонденции: lhayot_i@yahoo.com
У. Х. Аван: usman.awan@fao.org; М. Султанов: s.murod@mail.ru; А. Акрамханов: a.akramkhanov@cgiar.org; К. Джумабоев: k.jumaboev@cgiar.org; К. Конрад: christopher.conrad@uni-wuerzburg.de; Д. Ламерс: j.lamers(at)uni-bonn.de.
https://doi.org/10.29258/CAJWR/2019-R1.v5-2/111-127.rus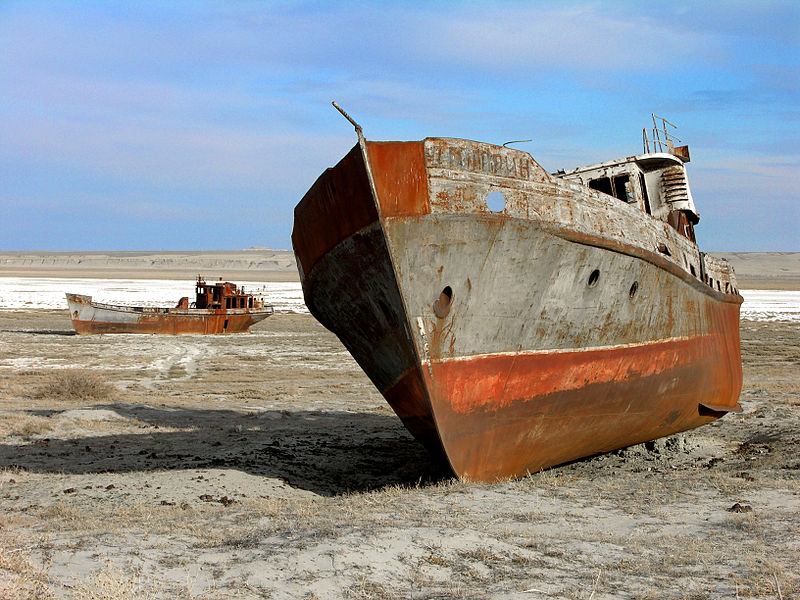
Аннотация
Точная оценка засоления почв является важным шагом для принятия мер по смягчению последствий деградации сельскохозяйственных земель. С целью оценки засоления широко используются инструменты дистанционного зондирования (ДЗ), однако при этом информация о точности такой оценки отсутствует. Оценка засоления на основе ДЗ в Хорезмской области позволяет получить значение надежности прогноза, имеющее слабую (R2=0,15–0,29) связь между картами засоления, полученными с помощью ДЗ и интерполяцией значений электромагнитной индукции, полученной с помощью инструмента EM38 в фазу роста растений, и более высокую корреляцию (R2=0,35–0,56) в период после орошения. Моделирование с помощью гидрологической модели HYDRUS-1D на слабозасоленных, среднезасоленных и сильнозасоленных участках на различной глубине почв показало, что в результате орошения соли перемещаются с верхних в более глубокие слои, а в период, когда орошение не проводится, эти соли вновь появляются в верхнем профиле почв. В конце периода орошения соли постепенно и стабильно накапливались в верхних слоях почв. Сочетание методов ДЗ с цифровым моделированием позволяет лучше понять динамику засоления по сравнению с применением этих подходов по отдельности. Результаты данного анализа могут представлять интерес для фермеров и лиц, принимающих решения, поскольку сочетание вышеуказанных методов позволит обеспечить лучшее планирование и управление ресурсами.
Скачать публикациюДля цитирования: Ибрахимов, М., Халид, У. and Султанов, М. (2019) ‘Сочетание подходов дистанционного зондирования и моделирования для оценки засоления почв орошаемых территорий бассейна Аральского моря’, Центральноазиатский журнал исследований водных ресурсов, 5(2), pp. 111–127. doi: 10.29258/CAJWR/2019-R1.v5-2/111-127.rus.
For citation: et al. (2020) ‘Sochetanie podhodov distancionnogo zondirovanija i modelirovanija dlja ocenki zasolenija pochv oroshaemyh territorij bassejna Aral’skogo morja [Combining remote sensing and modeling approaches to assess soil salinity in irrigated areas of the Aral Sea Basin]’, Central Asian Journal of Water Research, 5(2), pp. 111–127. doi: 10.29258/CAJWR/2019-R1.v5-2/111-127.rus [in Russian]
Список литературы
- Abbas, A., Khan, S., Akbar, S., Hanjra, M.A. and Hussain, N., 2013. Characterizing Soil Salinity in Irrigated Agriculture using a Remote Sensing Approach. Physics and Chemistry of the Earth, Vol. 55–57, pp. 43-52. Available at: https://doi.org/10.1016/j.pce.2010.12.004.
- Akramkhanov, A., Martius, C., Park, S. J., and Hendrickx, J. M. H., 2011. Environmental factors of spatial distribution of soil salinity on flat irrigated terrain. Geoderma, Vol. 163, No 1–2, pp. 55–62.
- Akramkhanov, A., Kuziev, R., Sommer, R, Martius, C., Forkutsa, O. and Massucati, L., 2012. Soils and Soil Ecology in Khorezm.
- Allen, R.G., Pereira, L.C., Raes, D. and Smith, M., 1998. Crop evapotranspiration. Guidelines for computing crop water requirements, pp. 1-300 FAO Irrigation and Drainage paper 56. FAO, Rome.
- Awan, U. K., Tischbein, B., Conrad, C., Martius, C. and Hafeez, M., 2011. Remote Sensing and Hydrological Measurements for Irrigation Performance Assessments in a Water User Association in the Lower Amu Darya River Basin. Water Resources Management, Vol. 25, No 10, pp. 2467–2485.
- Bannari, A., Guedon, A. M., El-Harti, A., Cherkaoui, F. Z. and El-Ghmari, A., 2008. Characterization of Slightly and Moderately Saline and Sodic Soils in Irrigated Agricultural Land using Simulated Data of Advanced Land Imaging (EO-1) Sensor. Communications in Soil Science and Plant Analysis, Vol. 39, No 19, pp. 2795–2811. Available at: https://doi.org/10.1080/00103620802432717.
- Bastiaanssen, W.G.M., Allen, R.G., Droogers, P., D’Urso, G., Steduto, P., 2007. Review: twenty-five years modeling irrigated and drained soils: state of the art. Agricultural Water Management, Vol. 92, pp. 111–125. Available at: https://doi.org/10.1016/j.agwat.
- Bucknall, J., Klytchnikova, I., Lampietti, J., Lundell, M., Scatasta, M. and Thurman, M., 2003. Irrigation in Central Asia: social, economic and environmental considerations. World Bank, Washington, DC, 52p.
- Conrad, C., Schorcht, G., Tischbein, B., Davletov, S., Sultonov, M. and Lamers, J. P. A., 2012. Agro-Meteorological Trends of Recent Climate Development in Khorezm and Implications for Crop Production.
- Dwivedi, R.S., and Rao, B.R.M., 1992. The selection of the best possible Landsat TM band combination for delineating salt-affected soils. International Journal of Remote Sensing, Vol. 13, pp. 2051–2058.
- Farifteh, J., Farshad, A. and George, R. J., 2005. Assessing Salt-Affected Soils Using Remote Sensing, Solute Modelling, and Geophysics. Geoderma, Vol. 130, No 3-4, pp. 191-206. Available at: http://dx.doi.org/10.1016/j.geoderma. 2005.02.003.
- FAO, 2000. FAO Global information and early warning system on food and agriculture. Special report: FAO/WFP crop and food supply assessment mission to the Karakalpakstan and Khorezm regions of Uzbekistan. Rome: FAO, 2000.
- FAO, 2003. Agriculture, Food and Water. ISBN 92-5-104943-2
- Forkutsa, I., Sommer, R., Shirokova, Y., Lamers, J. P. A., Kienzler, K., Tischbein, B., Martius, C. and Vlek, P. L. G., 2009. Modeling irrigated cotton with shallow groundwater in the Aral Sea Basin of Uzbekistan: II. Soil salinity dynamics, Irrigation Science, Vol. 27, pp. 319–330.
- Furby, S., Caccetta, P. and Wallace, J., 2010. Salinity monitoring in Western Australia using remotely sensed and other spatial data. Journal of Environmental Quality, Vol. 39, pp. 16–25.
- Golovina, N. N., Minskiy, D., Pankova, Y. and Solovyev, D. A., 1992. Automated air photo interpretation in the mapping of soil salinization in cotton-growing zones. Mapping Sciences and Remote Sensing, Vol. 29, pp. 262– 268.
- Hunt, G., Salisbury, J. and Lenhoff, C., 1972. Visible and near infrared spectra of minerals and rocks: V. Halides, phosphates, arsenates, venadates and borates. Modern Geology, Vol. 3, pp. 121– 132.
- Ibrakhimov, M., Khamzina, A., Forkutsa, I., Paluasheva, G., Lamers, J. P. A., Tischbein, B., Vlek, P. L. G. and Martius, C., 2007. Groundwater table and salinity: Spatial and temporal distribution and influence on soil salinization in Khorezm region (Uzbekistan, Aral Sea Basin). Irrigation and Drainage Systems, Vol. 21, pp. 219–236. Available at: https://doi.org/10.1007/s10795-007-9033-3.
- Lhissou, R., El Harti, A. and Chokmani, K., 2014. Mapping soil salinity in irrigated land using optical remote sensing data. Eurasian Journal of Soil Science, Vol. 3, No 2, pp. 82–88.
- Marquardt, D. W., 1963. An algorithm for least-squares estimation of nonlinear parameters. SIAM Journal on Applied Mathematics., Vol. 11, pp. 431-441.
- Metternicht, G.I. and Zinck, J. A., 2003. Remote sensing of soil salinity: potentials and constraints. Remote Sensing of Environment, Vol. 85, pp. 1–20.
- Metternicht, G. and Zinck, A., 2008. Remote Sensing of Soil Salinity: Impact on Land Management. CRC Press. Available at: http://dx.doi.org/10.1201/9781420065039.
- Metternicht, G., 1998. Analysing the relationship between ground based reflectance and environmental indicators of salinity processes in the Cochabamba Valleys (Bolivia). International Journal of Ecology and Environmental Sciences 24, 359– 370.
- Mulder, V. L, de Bruin, S., Schaepman, M. E. and Mayr, T. R., 2011 The use of remote sensing in soil and terrain mapping—a review. Geoderma, Vol. 162, No 1–2, pp. 1–19.
- Oster, J. D. and Rhoades, J. D., 1990. Steady state root zone salt balance. In: Tanji, K. K. (ed.), Agricultural salinity assessment and management manual. ASCE, New York, pp. 469–481.
- Rao, B., Sankar, T., Dwivedi, R., Thammappa, S., Venkataratnam, L., Sharma, R. and Das, S., 1995. Spectral behaviour of salt-affected soils. International Journal of Remote Sensing, Vol. 16, pp. 2125– 2136.
- Singh, R., van Dam, J. C. and Jhorar, R.K., 2003. Water and salt balances at farmer fields. In van Dam, J. C. and Malik, R. S. (eds.), Water Productivity of irrigated crops in Sirsa district, India, integration of remote sensing, crop and soil models and geographical information systems. Wageningen University, Department of Water Resources, The Netherlands, p. 41-58.
- Vogel, T. and Cislerova, M., 1988. On the reliability of unsaturated hydraulic conductivity calculated from the moisture retention curve. Transport in porous media, Vol. 3, pp. 1-15.
- Šimunek, J., Šejna, M., Saito, H., Sakai, M. and van Genuchten, M.Th., 2008. The HYDRUS-1D Software Package for Simulating the Movement of Water, Heat, and Multiple Solutes in Variably Saturated Media, Version 4.0, HYDRUS Software Series 3. Department of Environmental Sciences, University of California Riverside, Riverside, CA, USA, pp. 315.
HYDRUS-1D, Аральское море, засоление почв, моделирование солепереноса, орошение